With global cloud spending projected to hit $1.35 trillion by 2027, it is clear that many businesses and even individuals are either using or investing in cloud computing. Within this growing market, cloud GPUs have become a key area of investment.
The numbers speak volumes: the GPU as a Service (GPUaaS) market, valued at $3.23 billion in 2023, is expected to skyrocket to $49.84 billion by 2032, and it’s mostly due to the high demand for AI and machine learning applications. The growth trajectory is clear—but does that mean renting cloud GPUs is the right financial move for your business?
This article breaks it all down. We’ll discuss real-world cost comparisons and give strategic insights on how to navigate the economics of cloud GPUs easily.
Source: NVIDIA
If cost-effective cloud solutions are what you need, CUDO Compute provides that, and you can get started with just a few clicks. Click here to begin.
When to rent a cloud GPU
Cloud GPUs offer significant benefits, but they’re not a one-size-fits-all solution. To determine if renting is the right choice for you, consider the following scenarios where it shines:
1. Short-term projects and peak demand
- Project-based needs: For short-term projects requiring intensive GPU power—like training machine learning models or rendering complex animations—renting eliminates the upfront cost and long-term commitment of buying hardware.
- Handling demand spikes: If your workload fluctuates, cloud GPUs provide the flexibility to scale up during peak periods without investing in additional hardware that might sit idle once demand subsides.
2. Experimentation and innovation
- Exploring new technologies: Cloud platforms let you experiment with different GPUs and configurations without high upfront costs—ideal for testing AI algorithms, game development, or research initiatives.
- Proof of concept: Before committing to expensive on-premise infrastructure, you can use cloud GPUs to validate your ideas, assess feasibility, and demonstrate their potential.
3. Accessibility and collaboration
- Democratizing GPU access: Cloud services provide access to powerful GPUs for individuals, startups, and researchers who might lack the resources to purchase and maintain their own hardware.
- Enabling seamless teamwork: Cloud environments support easy collaboration, allowing teams to share resources, work on projects simultaneously, and access data from anywhere in the world.
4. Reduced IT overhead
- Offloading Maintenance: Renting allows you to hand over hardware maintenance, software updates, and security to the cloud provider, freeing your team to focus on high-value tasks.
- Simplified Infrastructure: With no need for physical space, cooling systems, or power infrastructure, cloud GPUs minimize operational complexity and associated costs.
5. Cost-effectiveness for specialized workloads
- Tailored solutions: Many providers offer optimized GPU instances for specific workloads, such as deep learning or scientific computing. These options can deliver better performance and cost efficiency than general-purpose GPUs.
By evaluating your specific requirements and weighing these factors, you can make a strategic decision that aligns with your business goals, maximizes performance, and minimizes unnecessary costs.
Cost of renting cloud GPUs
When considering the cost of renting cloud GPUs, the decision goes beyond a simple price tag. Workload requirements, provider pricing structures, and hidden expenses can significantly influence your total cost.
Let’s break down the key elements, including a practical scenario, to help you understand and control your costs.
1. Hourly vs. reserved pricing (Including bare metal and clusters)
- On-demand instances: Many cloud providers offer hourly or pay-as-you-go pricing, making it ideal for short-term projects. For example, renting an NVIDIA A100 on CUDO Compute costs $1.50 per hour on demand.
On-demand pricing is helpful for users who require flexibility and need resources for unpredictable workloads.
- Reserved instances: If you expect consistent usage, reserved or long-term contracts can save you 40–60% compared to on-demand pricing. These savings make reserved instances a smart choice for ongoing projects like AI training, HPC workflows, or large-scale simulations, where consistent performance is key.
- Bare metal servers: For users who need dedicated resources without the overhead of virtualization, bare metal servers offer high performance and full hardware control. For example:
Renting a bare metal server with 8 NVIDIA A100 GPUs costs $12.80 per hour on CUDO Compute. You should use bare metal servers when you need predictable performance, such as for real-time AI inference or rendering tasks.
- GPU clusters: GPU clusters provide scalable compute power for large-scale workloads, such as training deep learning models on massive datasets or running simulations across multiple nodes.
Pricing for clusters depends on the number of GPUs and interconnect speed you need. For example, depending on the network bandwidth and GPU type, a H100 16-GPU cluster on CUDO Compute might cost $30–$50 per hour. GPU clusters are especially useful for enterprises conducting multi-GPU parallel processing or researchers running distributed AI training.
By carefully analyzing your workload requirements, you can select the pricing model and infrastructure—on-demand, reserved, bare metal, or clusters—that best fits your project’s needs and budget.
2. Pricing by GPU type
Not all GPUs are priced equally. Advanced GPUs like NVIDIA H200 or H100 cost more than older options like the V100 or A4000. Matching your workload with the right GPU will help you avoid overpaying for unnecessary performance.
If you need to learn what some of these GPUs can do and which you should use, check out our blog. We’ve compared the A100 vs H100, H100 vs H200, and more. You can also check our AI GPU benchmarks.
3. Storage and data transfer costs
GPU rental is only part of the equation. Cloud providers often charge separately for associated services like storage. For example, storing 1TB of training data might cost you around $5 per month for standard storage or more for high-speed SSD options.
4. Hidden costs and considerations
- Idle resources: Forgetting to shut down instances when not in use can result in unexpected expenses. Monitoring tools and automated workflows can help mitigate this risk.
- Scaling costs: Costs can escalate quickly if you need to scale up multiple GPUs simultaneously. Assess whether your budget aligns with peak demand scenarios.
You can make smarter decisions about renting cloud GPUs by evaluating your needs and running scenarios like the one above. Using a real-world example, let’s consider a scenario of what this might cost you and how you can save costs.
Scenario: AI model training cost breakdown
A company needs to train a deep-learning model for computer vision. The task requires 1,000 GPU hours and roughly 1TB of storage for their model and data. Here's a cost breakdown for three cloud providers using 8 NVIDIA H100 GPUs. We’ll consider Azure, AWS, and CUDO Compute.
Read our comparison of AWS VS CUDO compute here.
This is their base setup:
Platform | vCPUs | Memory (GiB) | GPU | Instance |
AWS | 192 | 2048 | 8x H100 | p5.48xlarge |
Azure | 96 | 1900 | 8x H100 | ND96isr H100 v5 |
AWS and Azure have very similar pricing structures for their H100 instances. Their configuration is in the above table, which we will set up on CUDO Compute. Depending on location, the Azure instance costs $127 per hour on average, and the AWS instance costs $116.4941 per hour on average. Although, for both providers, their least expensive location each costs $98 per hour.
To know the cost of a similar configuration on CUDO Compute, we must get each piece (GPU, vCPU, and storage) of the instance separately. It might seem more complicated, but this makes it easier because you are not stuck with a pre-made configuration, and you know precisely what you are paying for.
The cost of these per hour on CUDO Compute is $22.68. Here is the breakdown:
Compute | Value | Price per unit | Total | |
H100 | 8 | $2.45 | $19.60 | |
vCPU | 192 | $0.0021 | $0.40 | |
Memory (GB) | 2000 | $0.0013 | $2.60 | |
Storage (GB) | 1024 | $0.000077 | $0.079 | |
Total | $22.68 |
If the company is using the least expensive location for their AI model training, here is how much they’ll spend on each platform:
Platform | Cheapest location (PPH) | Training time (Hours) | Cheapest location training cost | |
AWS | $98.00 | 1000 | $98,000.00 | |
Azure | $98.00 | 1000 | $98,000.00 | |
CUDO Compute | $22.48 | 1000 | $22,682.05 |
* PPH = price per hour
If the company, for any reason, cannot use any of these locations, while the price will basically stay the same on CUDO Compute, the cost of their training on AWS and Azure will be as follows, 一assuming they are using the average-priced location:
Platform | Average location (PPH) | Training time (Hours) | Average location training cost | |
AWS | $116.49 | 1000 | $116,490.00 | |
Azure | $127.00 | 1000 | $127,000.00 | |
CUDO Compute | N/A | 1000 | N/A |
If the company is flexible and can use spot instances, it could save over 50% compared to on-demand rates on AWS and Azure. However, spot instances risk being interrupted, so they might not suit all use cases. Reserved instances offer a middle ground with their predictability and discounted rates, but they are usually for projects lasting from a year and above.
If the same company opts for on-premise infrastructure, the costs would include the hardware and associated operational expenses, as an NVIDIA H100 GPU could cost over $30,000. Here's an estimated breakdown for an 8-GPU server:
Side Note: We can’t give you the exact cost of everything as the price will differ depending on your location, but we will give you an estimate as close as possible.
1. GPUs: You want 8 NVIDIA H100 GPUs. At $30,970.79 each, that's 8 * $30,970.79 = $247,766.32
2. CPUs: 96 CPUs is a lot. And since you’re using a high-end GPU server, you’ll likely use a multi-socket server with high core-count CPUs. Suppose you go with top-of-the-line AMD EPYC 9004 series CPUs with 96 cores each. A reasonable estimate for two of these CPUs is around $20,000 - $30,000 total. Let's say $25,000 for now.
3. Storage: 1TB of SSD storage is relatively inexpensive in the grand scheme of things. You can get high-end enterprise NVMe drives for around $500-$1000. Let's budget $1,000.
4. NVSwitch: NVIDIA NVSwitch is the key to connecting those 8 GPUs with 900 GB/s bandwidth. These are not sold separately and are integrated into NVIDIA DGX H100 systems. We'll have to factor in a significant cost for this.
5. The "Everything Else": This is where it gets trickier and where a lot of the cost will come from:
- Motherboard: You'll need a specialized motherboard designed for this many CPUs and GPUs with the proper PCIe lanes and support for NVSwitch. This could easily be $10,000+.
- RAM: With 96 CPU cores, you'll want a LOT of RAM. Let's say 2TB of high-speed DDR5, which could be another $10,000 - $20,000.
- Power Supply: 8 H100 GPUs have a TDP of 700W each, plus the CPUs and other components. You'll need multiple redundant power supplies, likely in the 8-10kW range. This could be another $5,000 - $10,000.
- Cooling: This system will generate a massive amount of heat. You'll need a robust cooling solution, potentially liquid cooling, which adds significant cost.
- Chassis: A server chassis to house all this will be specialized and expensive.
- Networking: High-bandwidth networking is essential, so expect 100GbE or faster networking cards.
- Software and Licensing: Don't forget the cost of the operating system, drivers, and any specialized software.
Component | Estimated Price (USD) | Notes | ||
8 x NVIDIA H100 GPUs | $247,766.32 | Based on $30,970.79 per GPU | ||
96 x CPUs | $25,000 | Assuming dual high-core-count AMD EPYC 9004 series CPUs | ||
1TB SSD Storage | $1,000 | High-end NVMe drives | ||
Motherboard | $10,000+ | Specialized board for multiple CPUs and GPUs, NVSwitch support | ||
RAM | $10,000 - $20,000 | 2TB+ of high-speed DDR5 RAM | ||
NVSwitch | Included in DGX H100 | Integrated into NVIDIA DGX H100 systems | ||
Power Supply | $5,000 - $10,000 | Multiple redundant units, likely in the 8-10kW range | ||
Cooling | $5,000+ | Robust cooling solution, potentially liquid cooling | ||
Chassis | $5,000+ | Specialized server chassis | ||
Networking | $2,000+ | High-bandwidth networking cards (100GbE or faster) | ||
Software & Licensing | $5,000+ | Operating system, drivers, and any specialized software | ||
Total | $325,000 - $425,000+ | Very rough estimate. Actual cost may vary significantly. |
The above configuration is very similar to an NVIDIA DGX H100 system. You should consider exploring that option as it is more cost-effective and easier to manage. The NVIDIA DGX H100 could cost over $300,000.
When deciding between renting cloud GPUs and building an on-premise infrastructure, you need to look beyond hardware costs and consider ongoing operational expenses. Running physical servers comes with substantial electricity, cooling, and maintenance costs.
For instance, 8 NVIDIA H100 GPUs have a total power consumption of over 5.6kW, and factoring in CPUs, networking, and cooling, your power requirements could easily exceed 10kW. Depending on local electricity prices, that’s $1,000–$2,000 per month just to keep the system running.
Cooling solutions—especially for high-density setups—can add significantly to your power consumption and operating costs. Many companies opt for liquid cooling, which improves performance but requires a larger upfront investment and ongoing maintenance. Additionally, to manage an on-premise server, you'll need staffing or contracting IT professionals for hardware upkeep, software updates, and troubleshooting, which can cost another $500–$1,000 per month.
Over time, these costs can make on-premise infrastructure even more expensive than its initial cost. In contrast, cloud providers absorb these operational expenses, offering a more predictable and scalable cost structure, which allows companies to allocate their budgets more efficiently and focus resources on innovation and growth.
Carefully evaluating your workload type, project duration, and budget constraints, you can decide whether renting cloud GPUs or investing in on-premise infrastructure is better. For many, the flexibility and scalability of cloud GPUs offer significant cost and operational advantages.
If your company needs performance and scalability, try our enterprise solution. We also give you access to bare metal servers and scalable GPU clusters, with options tailored to your AI, HPC, and deep learning needs. Contact us to learn more.
Learn more: LinkedIn , Twitter , YouTube , Get in touch .
Continue reading
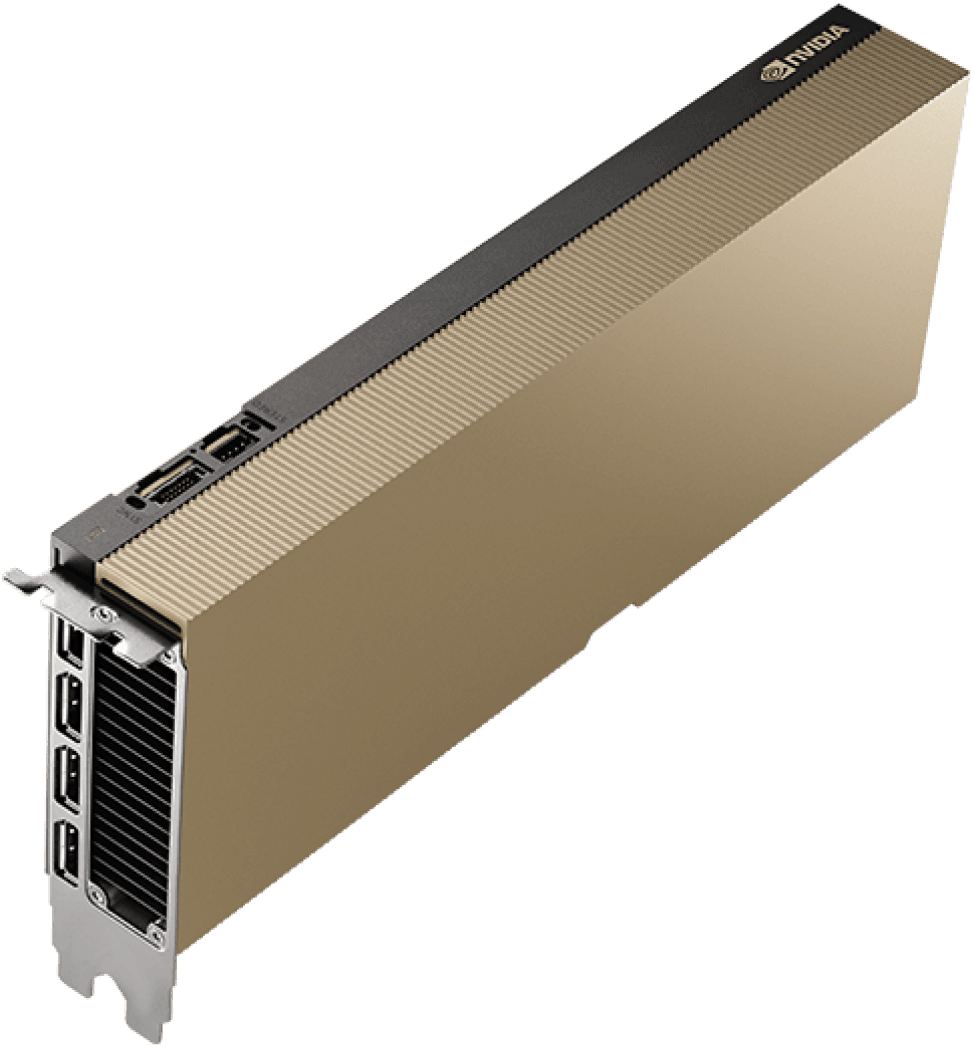
NVIDIA L40S's available from $1.42/hr
Starting from $0.75/hr
NVIDIA L40S's are now available on-demand
A cost-effective option for AI, VFX and HPC workloads. Prices starting from $0.75/hr
Subscribe to our Newsletter
Subscribe to the CUDO Compute Newsletter to get the latest product news, updates and insights.