In Deep Learning (DL), the role of graphics processing units (GPUs) can hardly be overstated. These powerful processors accelerate the complex mathematical computations required in deep learning tasks, enabling faster and more efficient model training and inference. With the exponential growth and demand for artificial intelligence (AI) applications, the need for high-performing GPUs has never been greater.
Two such GPUs making significant strides in the market are NVIDIA's A40 and A100. Both belong to NVIDIA's renowned Ampere architecture series and are engineered to accelerate AI workloads, pushing the boundaries of what's possible in deep learning.
This article compares these two GPUs, focusing on their deep learning performance. Whether users are data scientists striving to train more accurate models, AI researchers pushing the frontiers of machine learning, or tech enthusiasts curious about the latest hardware trends, this comparison will shed light on which GPU might best suit their needs.
Specifications of the NVIDIA A40 and A100
The NVIDIA A40 is a professional graphics card based on the Ampere architecture. It features 48GB of GDDR6 memory with ECC and a maximum power consumption of 300W.
Conversely, the NVIDIA A100, also based on the Ampere architecture, has 40GB or 80GB of HBM2 memory and a maximum power consumption of 250W to 400W2.
The NVIDIA A40 and A100 are high-performance graphics cards designed for data centres and professional applications built on NVIDIA's Ampere architecture. Let's compare their specifications:
NVIDIA A40
The NVIDIA A40 is a high-performance graphics card designed for data centre applications, utilising the NVIDIA Ampere architecture. It is specifically engineered to handle large datasets and complex computations, making it well-suited for AI research, data science, and high-performance computing.
Key Specifications and Features:
- Memory: The A40 has 48GB of GDDR6 memory, incorporating error-correcting code (ECC) to ensure reliability in data-intensive tasks.
- Core Configuration: It boasts 10,752 CUDA Parallel Processing cores, 336 NVIDIA Tensor Cores, and 84 NVIDIA RT Cores, which are designed for parallel processing and AI workloads.
- Memory Bandwidth and NVLink: The A40 offers a GPU memory bandwidth of 696 GB/s. It also supports NVIDIA NVLink, which provides a bidirectional speed of up to 112.5 GB/s and enhances interconnectivity between GPUs.
- Manufacturing and Transistors: Built on Samsung's 8nm process, the A40 features a die size of 628 mm² and contains 28.3 billion transistors, highlighting its advanced design and capability.
The A40 is tailored for demanding AI, data science applications, and high-performance computing environments. Its large memory, high computational power, and fast data transfer rates make it ideal for handling complex, data-heavy tasks. Its combination of ECC memory, high CUDA core count, and NVLink support positions it as a reliable choice for a range of computational tasks in professional and research settings.
NVIDIA A100
As discussed in our previous article, the NVIDIA A100 is a formidable graphics card designed for data centre applications, leveraging the NVIDIA Ampere architecture. It stands out as a leading solution in large-scale machine learning infrastructure. The A100 is available in two primary editions: one that utilises NVIDIA's high-performance NVLink network infrastructure and another based on the traditional PCIe interface. This versatility allows it to be integrated into various server environments.
Key Specifications and Features:
- Memory Options: The A100 comes with 40GB or 80GB of memory, catering to different computing needs.
- Architecture: It's based on the Ampere GA100 GPU and specifically optimised for deep learning workloads, making it one of the fastest GPUs for such tasks.
- Manufacturing Process: It is built on a 7 nm process by TSMC, features a die size of 826 mm², and packs 54,200 million transistors.
- Core Configuration: The A100 has 6,912 shading units, 432 texture mapping units, 160 ROPs, and 432 tensor cores, which are pivotal in accelerating machine learning applications.
- Memory Type and Bandwidth: It uses HBM2e memory, which doubles the memory capacity compared to the previous generation and offers over 2TB of memory bandwidth per second.
- Performance Capabilities: The A100 provides peak performance capabilities across various computing metrics, such as 9.7 TF for FP64, 19.5 TF for Tensor Cores in FP64, 312 TF for Tensor Cores in FP16/BFLOAT16, and up to 1,248 TOPS on Tensor Cores for INT4.
- MIG Technology: The Multi-Instance GPU (MIG) technology allows the A100 to be divided into up to 7 isolated GPU instances, enhancing its versatility in workload management.
- NVLink and NVSwitch: The third-generation NVLink in A100 enhances GPU scalability, performance, and reliability with a 600 GB/s total bandwidth, significantly higher than its predecessors.
The A100 is primarily intended for AI research, high-performance computing, and data science, where large-scale machine learning infrastructure is required. Its advanced features and capabilities make it particularly suitable for environments that require high throughput, low latency, and efficient parallel processing of complex computations.
The NVIDIA A100 represents the cutting edge in GPU technology for data centres, offering unparalleled performance in machine learning, AI inference, and high-performance computing tasks. Its versatile configurations, advanced memory, and core technologies make it a top choice for demanding computational workloads.
Comparative analysis of the A40 and A100
Compared to the NVIDIA A40, the A100 offers different memory configurations, higher bandwidth, advanced features like MIG technology and superior NVLink performance. While both are based on the Ampere architecture, the A100's larger die size, higher transistor count, and advanced memory type (HBM2e) position it for more intensive computational tasks, especially deep learning and AI. Here are some key differences:
- Architecture and Manufacturing Process: Both GPUs are based on the Ampere architecture. Still, the A100 is built using a smaller 7 nm process than the 8 nm process of the A40 and by different manufacturers (TSMC for A100 and Samsung for A40).
- Performance Cores: The A40 has a higher number of shading units (10,752 vs. 6,912), but both have a similar number of tensor cores (336 for A40 and 432 for A100), which are crucial for machine learning applications.
- Memory: The A40 comes with 48 GB of GDDR6 memory, while the A100 has 40 GB of HBM2e memory. The A100's memory has a significantly wider interface and higher bandwidth.
- Target Applications: Both are designed for AI, data science, and high-performance computing, but the A100's larger die size and transistor count, along with its higher memory bandwidth, suggest it might be more suitable for extremely large-scale computations.
The NVIDIA A40 and A100 are advanced GPUs built on the same architecture, and they have different specifications tailored to their respective target applications in professional and data centre environments. The A100 is positioned for more intensive computational tasks, given its larger die size, higher transistor count, and superior memory bandwidth.
What are Deep Learning GPU benchmarks?
Deep learning GPU benchmarks are tests conducted to measure and compare the performance of different GPUs (Graphics Processing Units) in deep learning tasks. These tasks often involve complex computations, requiring high processing power. Benchmarks typically focus on key metrics such as processing speed (TFLOPS), memory bandwidth (GB/s), and power efficiency (TFLOPS/Watt). By comparing these figures, users can decide which GPU best suits their specific needs for deep learning applications.
Benchmarks and performance metrics
Benchmarking in the context of deep learning involves measuring the performance of specific hardware using a set of relevant metrics. This process allows for comparing performance across different systems or configurations, providing valuable insights for organisations to develop plans and make informed decisions.
Key metrics for deep learning benchmarking include:
- Processing Speed: This metric, often measured in teraflops (TFLOPS), indicates how quickly a GPU can process data, representing the number of trillion floating-point operations it can perform per second. This is particularly crucial in deep learning applications where large volumes of data need to be processed swiftly.
- Memory Bandwidth: This refers to the rate at which data can be read from or stored in the semiconductor memory by the processor. Higher memory bandwidth allows for faster data transfer, which is essential when handling large datasets in deep learning.
- Power Efficiency: This metric measures the amount of work a GPU can do per unit of power consumed. Given the high computational demands of deep learning, power efficiency becomes critical in determining the overall cost and feasibility of running intensive AI workloads.
These are some key performance benchmarks for the A140 and A100:
The results of these benchmarks can be presented in a comparative format, such as charts or graphs. The visualisations below provide a clear and concise overview of the relative performance of different GPUs, aiding users in selecting the most suitable GPU for their specific needs.
These benchmarking metrics will be pivotal in determining which GPU offers superior performance for deep learning applications.
Based on these, it is clear that the NVIDIA A100 outperforms the A40 in several key areas: it has more than double the VRAM bandwidth, lower power consumption (TDP), and significantly more tensor cores. These factors make the A100 a superior choice for tasks that require high computational power, such as deep learning.
However, the A40 does have its advantages. It has a higher boost clock, more VRAM, and more rendering cores, which could make it a better choice for tasks that require high memory capacity and fast rendering, such as 3D modelling.
In terms of cost efficiency, the A40 is higher, which means it could provide more performance per dollar spent, depending on the specific workloads.
Ultimately, the best choice will depend on your specific needs and budget.
Deep Learning performance analysis for A100 and A40
Deep learning tasks can broadly be categorised into two main types: training and inference. Training involves using large datasets to train a model, while inference uses the trained model to predict new data. The performance of GPUs like the NVIDIA A40 and A100 in these tasks is paramount. This is how they perform in these tasks:
Training
The NVIDIA A40, with its 48GB GDDR6 memory and high processing speed, is well-equipped for handling the large datasets typically associated with deep learning training. It can efficiently process complex computations, making it an excellent choice for AI research, data science, and high-performance computing.
The NVIDIA A100, on the other hand, is designed for the most demanding AI and high-performance computing workloads. Thanks to its advanced features and powerful capabilities, it's known for its exceptional performance in AI training tasks.
Inference
Regarding inference, both GPUs excel due to their high processing speeds and memory bandwidths. These features allow them to quickly predict new data using trained models, making them ideal for real-time applications.
Different neural network models and data sizes can affect GPU performance. For instance, convolutional neural networks (CNNs) are commonly used in image processing and require high computational power, which both the A40 and A100 can provide. Recurrent neural networks (RNNs), used for sequential data like time series or natural language, also perform well on these GPUs due to their high memory bandwidth.
Larger datasets require more memory and processing power. Both GPUs have ample memory to handle large datasets effectively. However, the specific performance may vary based on the complexity of the data and the specific requirements of the deep learning task.
The NVIDIA A40 and A100 GPUs offer robust performance for deep learning applications. The A40 stands out for its ample memory and high processing speed, making it a reliable choice for AI research, data science, and high-performance computing. The A100, designed for the most demanding AI workloads, excels in training tasks due to its advanced features and powerful capabilities.
The choice between the two will depend on user needs and project requirements. For instance, organisations with demanding AI workloads might opt for the A100 because of its superior performance, while those needing a balanced GPU for a range of tasks may find the A40 more suitable. Ultimately, both GPUs represent solid investments for anyone leveraging deep learning for their projects.
Both the impressive A100 and the reliable A40 are now available on CUDO Compute. Whether tackling AI workloads, data science tasks, or complex computations, CUDO Compute offers the performance and pricing flexibility you need with a wide array of high-performance GPUs. Get in touch to experience superior performance for your projects today!
About CUDO Compute
CUDO Compute is a fairer cloud computing platform for everyone. It provides access to distributed resources by leveraging underutilised computing globally on idle data centre hardware. It allows users to deploy virtual machines on the world’s first democratised cloud platform, finding the optimal resources in the ideal location at the best price.
CUDO Compute aims to democratise the public cloud by delivering a more sustainable economic, environmental, and societal model for computing by empowering businesses and individuals to monetise unused resources.
Our platform allows organisations and developers to deploy, run and scale based on demands without the constraints of centralised cloud environments. As a result, we realise significant availability, proximity and cost benefits for customers by simplifying their access to a broader pool of high-powered computing and distributed resources at the edge.
Continue reading
Real-world benchmarks demonstrating performance variances across different GPU cloud infrastructures
11 min read
Choosing a GPU cloud provider in 2025: A proven evaluation checklist
25 min read
How to select the right GPU for your AI workload
18 min read
What is the cost of training large language models?
26 min read
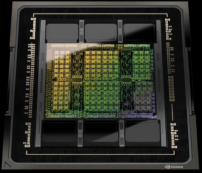
High-performance cloud GPUs